Keynote Speakers
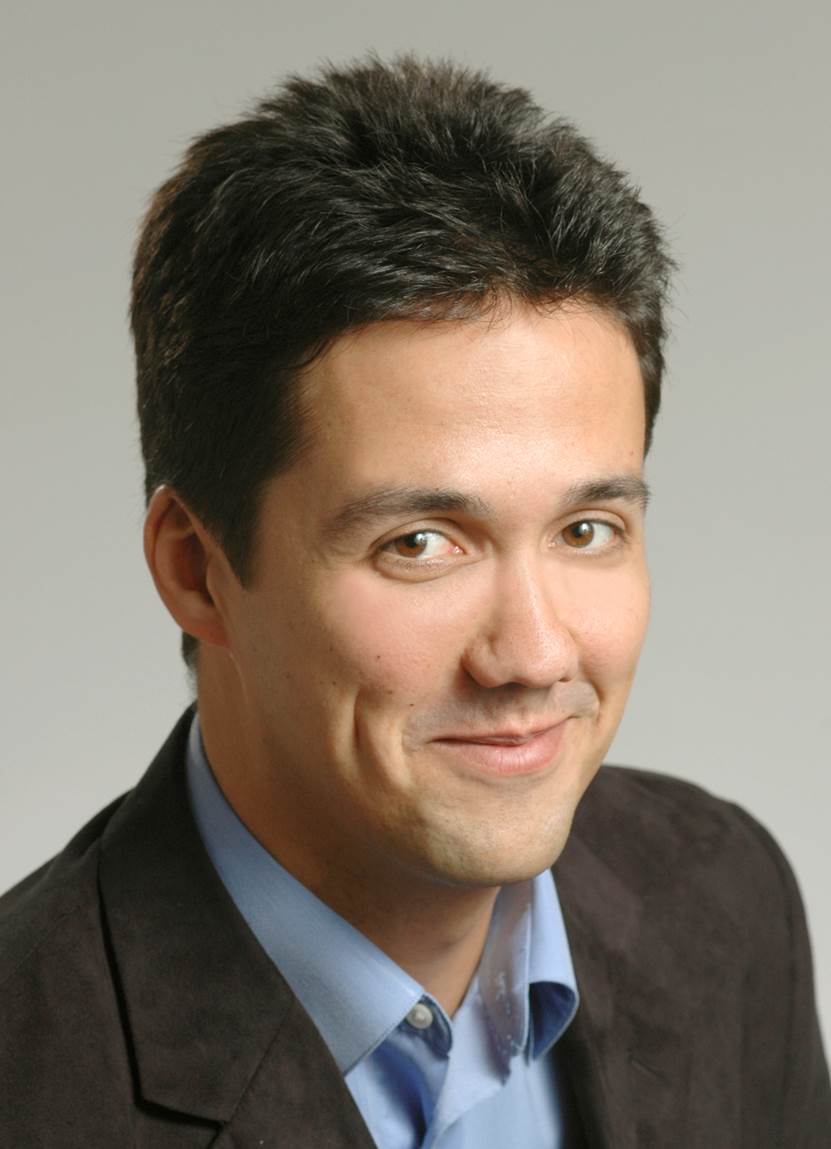
Prof. Jose M. Carmena
UC Berkeley
Department of Electrical Engineering and Computer Sciences
Helen Wills Neuroscience Institute
Title: Advances in Brain-Machine Interface Systems
Abstract:
Brain-machine interfaces (BMIs) is a novel technology that holds great potential to aid large numbers of people with sensory, motor and cognitive disabilities. BMIs provide also a framework for examining basic neuroscience questions, especially those related to the understanding of how neural plasticity relates to the acquisition and consolidation of neuroprosthetic skills, i.e. accurate, readily-recalled control of disembodied actuators irrespective of natural physical movement. In this talk I will postulate that achieving skillful, natural control of a multi-degree-of-freedom prosthetic device will entail synergizing two different types of adaptation processes: natural (brain plasticity) and artificial (decoder adaptation), as well as providing realistic sensory feedback from the prosthetic device. I will present recent work from our laboratory showing that 1) neuroplasticity facilitates consolidation of neuroprosthetic motor skill in a way that resembles that of natural motor learning; 2) corticostriatal plasticity is necessary for neuroprosthetic skill learning, and 3) closed-loop decoder adaptation (CLDA) techniques can expedite the learning process by adapting the decoder parameters during closed-loop BMI operation (i.e., while the subject is using the BMI). We believe that BMI systems capable of exploiting both neuroplasticity and CLDA will be able to boost learning, generalize well to novel movements and environments, and ultimately achieve a level of control and dexterity comparable to that of natural arm movements.
Short Biography
Jose M. Carmena is Professor of Electrical Engineering and Neuroscience at the University of California-Berkeley, and Co-Director of the Center for Neural Engineering and Prostheses at UC Berkeley and UCSF. His research program in neural engineering and systems neuroscience is aimed at understanding the neural basis of sensorimotor learning and control, and at building the science and engineering base that will allow the creation of reliable neuroprosthetic systems for the severely disabled. Dr. Carmena received the B.S. and M.S. degrees in electrical engineering from the Polytechnic University of Valencia (Spain) in 1995 and the University of Valencia (Spain) in 1997. Following those he received the M.S. degree in artificial intelligence and the Ph.D. degree in robotics both from the University of Edinburgh (Scotland, UK) in 1998 and 2002 respectively. From 2002 to 2005 he was a Postdoctoral Fellow at the Department of Neurobiology and the Center for Neuroengineering at Duke University (Durham, NC). He is senior member of the IEEE (RA, SMC and EMB societies), Society for Neuroscience, and the Neural Control of Movement Society. Dr. Carmena has been the recipient of the Bakar Fellowship (2012), the IEEE Engineering in Medicine and Biology Society Early Career Achievement Award (2011), the Aspen Brain Forum Prize in Neurotechnology (2010), the National Science Foundation CAREER Award (2010), the Alfred P. Sloan Research Fellowship (2009), the Okawa Foundation Research Grant Award (2007), the UC Berkeley Hellman Faculty Award (2007), and the Christopher Reeve Paralysis Foundation Postdoctoral Fellowship (2003). More information of Prof. Carmena can be found: www.eecs.berkeley.edu/~carmena.
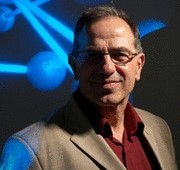
Prof. Nikola Kasabov
Auckland University of Technology
Knowledge Engineering and Discovery Research Institute
Title: Spiking Neural Networks and Spatio-Temporal Data Machines: Methods, Systems, Applications
Abstract:
All areas of science and human activities nowadays depend more and more on efficient and effective data analysis, modelling and event prediction. It has become obvious that the big data challenges, especially when we have to deal with massive temporal or spatio/spectro-temporal data (SSTD), could not be addressed properly with the use of traditional statistical and machine learning methods, nor with the use of traditional computational architectures. Spiking neural networks (SNN), combined with brain infortion principles for large scale information processing, offer hope to address this problem. The talk introduces a new computational architecture, called here spatio-temporal data machine (STDM), which uses brain information processing principles, such as: integrated and distributed memory and information processing among thousands an millions of spiking neurons and their connections; spatio-temporal learning rules; massive parallel computation; learning time and space interactions from multiple sources of data; predictive data processing; dynamic clustering and visualization; dynamic information exchange; model interpretation and knowledge extraction, and other, and doing all this in an energy economical way. The talk describes the details of the first implementation of a STDM, called NeuCube, developed in the author’s lab KEDRI. NeuCube is also a STDM development system that makes it possible to develop applications across domain areas using various types of SSTD. It includes modules for data encoding, unsupervised learning, supervised classification and regression, visualization, pattern discovery, model optimization. It has been implemented on both von Neumann computers and on neuromorphic systems. Some examples and demonstrations of STDM developed in NeuCube are: brain data models (EEG, fMRI, DTI); Brain computer interfaces (BCI) for cognitive games and rehabilitation; multiple sensor on-line data modelling and event prediction; dynamic personalized models for stroke and CVD prediction; seismic data modelling system for earthquake prediction; financial stock prediction; radio-astronomy data modelling. A student version of NeuCube development system, along with several data sets and exemplar applications, can be downloaded from: www.kedri.aut.ac.nz/neucube/.
Short Biography
Professor Nikola Kasabov is Fellow of IEEE, Fellow of the Royal Society of New Zealand and Distinguished Visiting Fellow of the Royal Academy of Engineering, UK. He is the Director of the Knowledge Engineering and Discovery Research Institute (KEDRI) at Auckland University of Technology (AUT). He is Chair of Knowledge Engineering in the School of Engineering, Computing and Mathematical Sciences. Kasabov holds adjunct positions at several other universities, such as: Shanghai Jiao Tong University, ETH/University of Zurich, RGU Aberdeen. Kasabov is a Past President and Governor Board member of the International Neural Network Society (INNS) and also of the Asia Pacific Neural Network Society (APNNS). Kasabov holds MSc and PhD from the TU Sofia, Bulgaria. His main research interests are in the areas of information sciences, neural networks, intelligent information systems, soft computing, bioinformatics, neuroinformatics, data mining. He has published more than 600 publications that include 15 books, 180 journal papers, 28 patents and numerous conference papers. He has extensive academic experience at various academic and research organizations in Europe and Asia, including: TU Sofia, University of Essex, University of Otago, University of Trento, TU Kaiserslautern. Prof. Kasabov has received several awards, including: the AUT Medal for 2015; APNNA ‘Outstanding Achievements Award’; INNS Gabor Award for ‘Outstanding contributions to engineering applications of neural networks’; EU Marie Curie Fellowship; Bayer Science Innovation Award; RSNZ Science and Technology Medal. He has supervised to completion 38 PhD students. He has been involved in the establishment of several spin-off companies at the University of Otago and AUT. More information of Prof. Kasabov can be found on the KEDRI web site: http://www.kedri.aut.ac.nz.
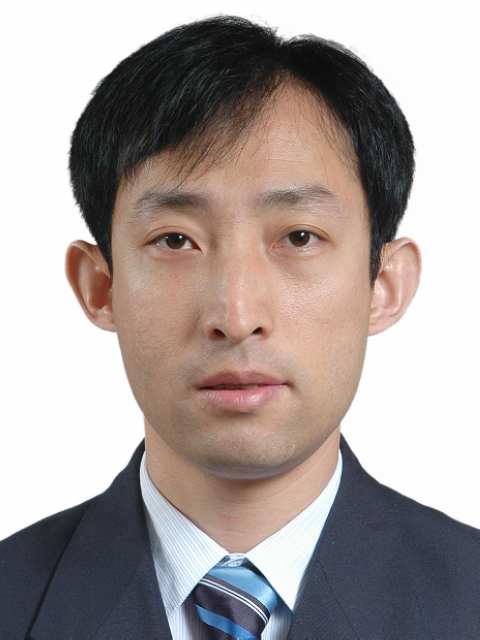
Prof. Huijun Gao
Harbin Institute of Technology
Research Institute of Intelligent Control and Systems
Title:
Networked Control Systems with Industrial Applications
Abstract:
In recent years, the analysis and synthesis of networked control systems (NCSs) have received increasing attention from both scientific and industrial communities. Compared with traditional point-to-point control systems, the main advantages of NCSs come from their low cost, their flexibility and easy re-configurability, their natural reliability and robustness to failure, and their adaptation capability. Consequently, NCSs have been finding applications in a broad range of areas such as power grids, water distribution networks, transportation networks, haptics collaboration over the Internet, mobile sensor networks, and so on. However, the introduction of communication channels in the control loop also brings some network-induced critical issues or constraints such as variable transmission delays, data packet dropouts, packet disorder, quantization errors, etc., which would significantly degrade the system performance or even destabilize the system in certain conditions. This talk will first introduce some elegant approaches to network-based control and estimation problems. Then, a novel two-layer network-based architecture for operational control of industrial processes will be discussed. It will be shown that under the proposed framework, the overall optimal operational control of networked industrial processes can be achieved.
Short Biography
Huijun Gao received his Ph.D. degree in control science and engineering from Harbin Institute of Technology, China, in 2005. He was a Research Associate with the Department of Mechanical Engineering, The University of Hong Kong, from November 2003 to August 2004. From October 2005 to October 2007, he carried out his postdoctoral research with the Department of Electrical and Computer Engineering, University of Alberta, Canada. Since November 2004, he has been with Harbin Institute of Technology, where he is currently a Professor and Director of the Research Institute of Intelligent Control and Systems. Prof. Gao’s research interests include network-based control, robust/intelligent control, robotics and their engineering applications. He is an IEEE Fellow, an IEEE SMC Distinguished Lecturer and received the IEEE IES David Irwin Early Career Award. He is Co-Editor-in-Chief of IEEE Transactions on Industrial Electronics and Associate Editor of Automatica, IEEE Transactions on Cybernetics, IEEE Transactions on Control Systems Technology, IEEE/ASME Transactions on Mechatronics etc. Prof. Gao is an IEEE Industrial Electronics Society (IES) Administration Committee (AdCom) member. He is a Thomson Reuters Highly Cited Researcher and was listed among the top 17 scholars in “The World’s Most Influential Scientific Minds” by Thomson Reuters, 2014. More information of Prof. Gao can be found: http://homepage.hit.edu.cn/pages/gaohuijun/2.